Learning from data is virtually universally useful. Master it and you’ll be welcomed nearly everywhere! —John Elder
The industry has seen a huge leap in the way it processes data. From being primarily focused on collecting data and deriving an actionable insight from it as seen a few decades ago, now it works mostly on the future predictions a.k.a. forecasting. Indeed, advancement.
Data prediction is like extrapolation; going back through past data, spotting a trend, and predicting where it will go in a few decades from now.
There is a reason why Predictive Analytics is one of the hottest trends amongst organizations, big or small. Apart from a greater understanding of different processes, indulging in predictive analytics helps businesses approach growth opportunities and risk factors better.
Like your secret weapon to success.
Hence, we build a ‘Predictive Model’. A model that we try to base our findings and expectations upon. A perfect model that could help guide our business to where you want it to be.
Only it isn’t an easy task. Having worked with 50+ successful predictive models till date, we would like to share a few tips and techniques that helped better our approach. Hope they help perfect yours too.
1. Determine the Performance of Your Current Model
The first step is to analyse where you must have gone wrong with your model set if you have one. First timers, stay tuned. We have actionable suggestions for you as well (refer point no. 2, 3, 4, 7, 8, and 9).
There are basically two types of glitches a Predictive Model usually experiences- high variance and high bias. High variance refers to a situation where your model performs exceptionally on the training set but is suffering too much on hold-out set. In the case of high bias, both training and test data sets don’t work in the favor of your model.
It’s better that you find out the lapse present in your model and correct or redo it in real time for an improved business foresight.
2. Avoid Common Mistakes
This technique best fits those building a Predictive Model for the first time.
It’s always better to take a BI/Big Data expert’s opinion before you begin framing a predictive model. Talk to people with experience in different versions and styles of predictive models, do your own research and understand what lapses and roadblocks you may face. Learning from successful models also could help you avoid any easy mistake.
Learning from successful models also could help you avoid any easy mistake.
3. Hire an Expert To Handle Your Project
Building a Predictive Model isn’t an easy task. You need a creative problem-solver on board, preferably an expert with years of experience in data handling, to take care of the extensive steps involved in predictive modeling- from collecting data, analysing them to utilising them to frame an actionable forecast.
4. Add More Data and Features
Adding data and features to the model helps decrease the degree of bias (or variance in the case of features), increasing the flexibility of the model.
But avoid adding features to your model if you are opting out of adding data and your original data set is pretty small in terms of data points.
5. Do Feature Selection
Almost opposite of the point above, this suggestion is for models with high features and not enough data points.
It drives variance down while adding to the flexibility of the model. The best way to do feature selection is to do it methodically, removing only the extra noisy and in-informative ones. In the case of models with enough data, you won’t even have to do the manual selection, the model will automatically handle the unimportant features.
In the case of models with enough data, you won’t even have to do the manual selection, the model will automatically handle the unimportant features.
6. Regularisation
If you can’t think of any other method, use regularisation. This way you can have a better control over the features involved in the model. Regularisation guides algorithm in using fewer yet apt features, and in implementing them smartly.
This way you can have a better control over the features involved in the model. Regularisation guides algorithm in using fewer yet apt features, and in implementing them smartly.
7. Bootstrap Aggregation (Bagging)
This method majorly focuses on reducing the high variance of the model.
A bunch of variations of the same model is created and a split test is performed on them. Thus, a working predictive model is chosen.
This process, while being thorough, is quite intensive on the computational front. It takes up a lot of memory.
8. Boosting
Boosting is a slow, complicated yet perfect method to reduce bias in a model. It involves creating successive training models that learn from the errors in the model preceding it.
Just like in the bagging technique, you may suffer from the high computation time and memory costs.
9. Reduce, Reuse, and Recycle
Make your models as sustainable as possible.
Reuse the cleansed data in different analyses. Reduce the burden on the model by automating report process. And recycle the knowledge obtained in future projects.
10. Change the Model (In case any of the above techniques don’t work :))
Banging your head (and your money) on a predictive model that doesn’t work is going to take you nowhere.
Be smart and change your model class. If you were working on a linear one, switch to the neutral network.
This change will help you know where you were lacking. There are times when a particular algorithm suits specific data sets, and your class might not fit that criterion. It is always better to look for a different model that suits your requirements.
The way to build a perfect predictive model isn’t an easy one. But always remember:
Predictive Model’s mission is to engineer solutions. As for the data employed and the insights gained, the tactic in play is: “Whatever works.”- Eric Siegel
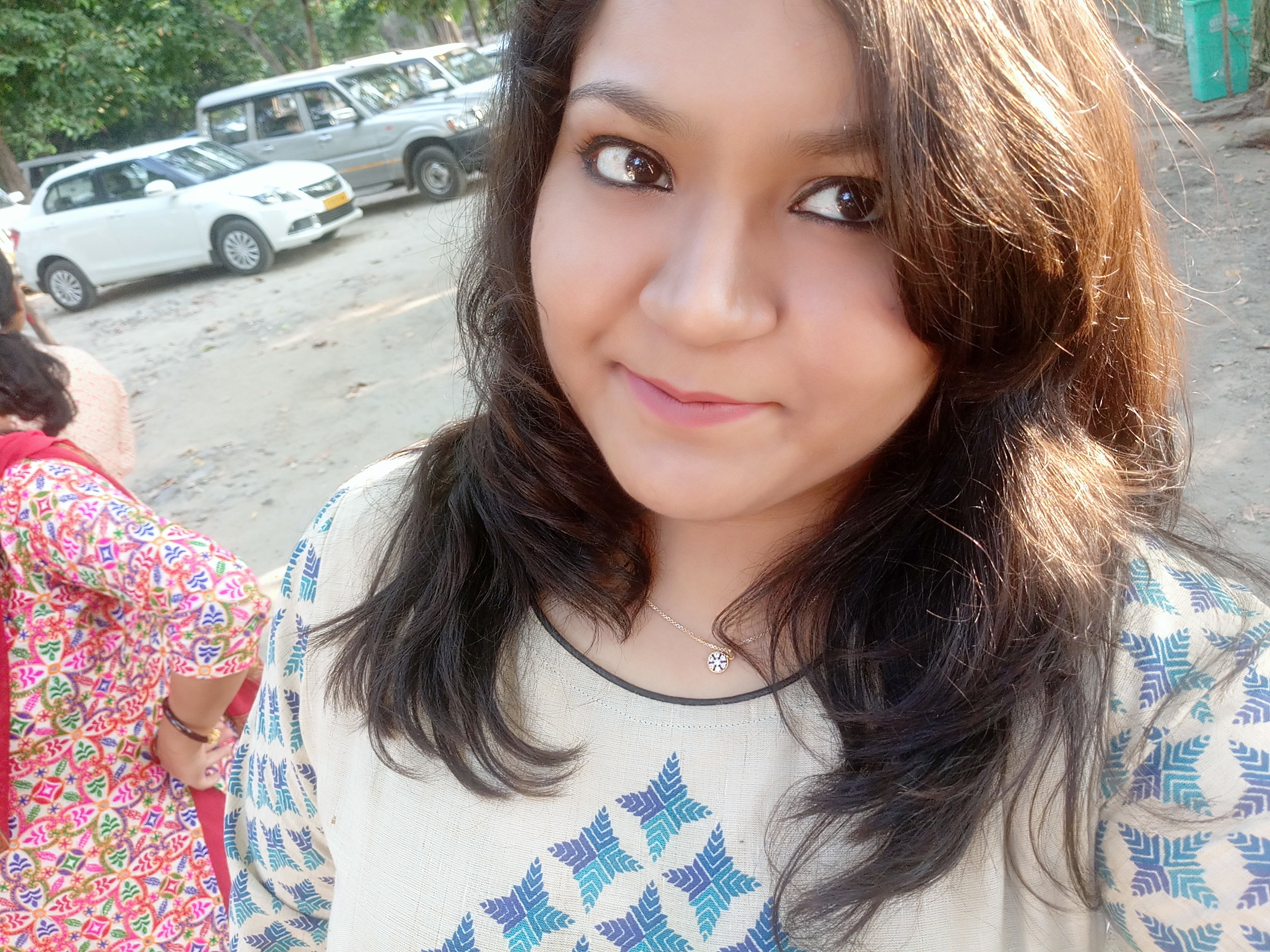
Written by Tanya Kumari
Tanya leads the Digital Marketing Team at Classic Informatics, a leading web development company . She is an avid reader, music lover and a technology enthusiast who likes to be up to date with all the latest advancements happening in the techno world. When she is not working on her latest article on agile team dynamics, you can find her by the coffee machine, briefing co-workers on the perks of living a healthy lifestyle and how to achieve it.